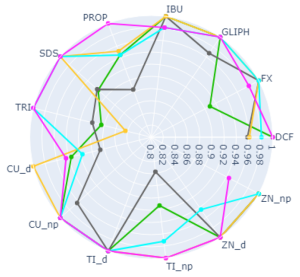
Rodrigues, Nuno M.; Batista, Joao E.; Mariano, Pedro; Fonseca, Vanessa; Duarte, Bernardo; Silva, Sara |
Simple Summary Our work is motivated by the increasing production of chemicals with environmentally harmful effects to our aquatic ecosystems. We show that it is possible to detect and distinguish the presence of several different emerging contaminants, using the photochemical responses of a microalgae species, which is among the most abundant phytoplankton group in the oceans. We use several machine learning and deep learning models that operate on chlorophyll fluorescence induction curves, which are composed of fluorescence values taken at different time steps from the microalgae exposure trials, achieving up to 97.65% accuracy when predicting the type of contaminant, and up to 100% in several cases when predicting the exposure concentration. Our results show the combination of these models with the fluorescence induction curves creates a powerful tool for ecotoxicity assessment, capable of classifying model organisms for their contaminant exposure, both in terms of type and concentration, opening new doors for toxicophenomics developments. Over recent decades, the world has experienced the adverse consequences of uncontrolled development of multiple human activities. In recent years, the total production of chemicals has been composed of environmentally harmful compounds, the majority of which have significant environmental impacts. These emerging contaminants (ECs) include a wide range of man-made chemicals (such as pesticides, cosmetics, personal and household care products, pharmaceuticals), which are of worldwide use. Among these, several ECs raised concerns regarding their ecotoxicological effects and how to assess them efficiently. This is of particular interest if marine diatoms are considered as potential target species, due to their widespread distribution, being the most abundant phytoplankton group in the oceans, and also being responsible for key ecological roles. Bio-optical ecotoxicity methods appear as reliable, fast, and high-throughput screening (HTS) techniques, providing large datasets with biological relevance on the mode of action of these ECs in phototrophic organisms, such as diatoms. However, from the large datasets produced, only a small amount of data are normally extracted for physiological evaluation, leaving out a large amount of information on the ECs exposure. In the present paper, we use all the available information and evaluate the application of several machine learning and deep learning algorithms to predict the exposure of model organisms to different ECs under different doses, using a model marine diatom (Phaeodactylum tricornutum) as a test organism. The results show that 2D convolutional neural networks are the best method to predict the type of EC to which the cultures were exposed, achieving a median accuracy of 97.65%, while Rocket is the best at predicting which concentration the cultures were subjected to, achieving a median accuracy of 100%.
Doi: 10.3390/biology10090932
Cited as: Rodrigues, Nuno M., João E. Batista, Pedro Mariano, Vanessa Fonseca, Bernardo Duarte, and Sara Silva. 2021. “Artificial Intelligence Meets Marine Ecotoxicology: Applying Deep Learning to Bio-Optical Data from Marine Diatoms Exposed to Legacy and Emerging Contaminants” Biology 10, no. 9: 932. https://doi.org/10.3390/biology10090932